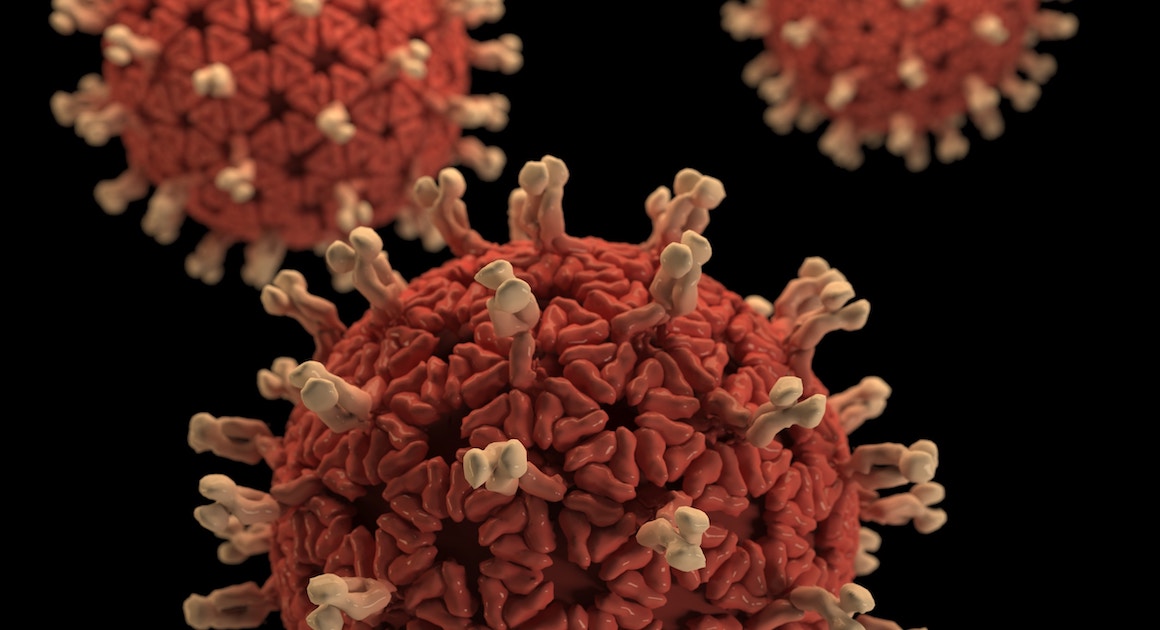
Coronavirus April 2020—Part 6 Evaluating Diagnostic Tests
This post includes math that may make your head spin, but we felt it was necessary to go through the statistics in detail to prove a crucial point, that antibody testing to SARS-CoV-2, the virus that causes the disease COVID-19, is not likely to tell us what we really want to know. We also have an update on the utility of wearing masks. If you’re less interested in how we got to our conclusions than you are in the conclusions themselves, feel free to skip to the BOTTOM LINE in each section and the CONCLUSION at the end. But first . . .
WHY DO WE NEED TO DO PROPER STUDIES At all?
The number of people clamoring for the use of interventions, like hydroxychloroquine or more recently remdesivir, to treat severe cases of COVID-19 based on anecdotal reports of effectiveness has been truly astonishing (though not nearly as astonishing as the number of physicians clamoring for the same thing). People have accused those of us arguing that we should avoid the use of interventions with only anecdotal evidence of being in favor of allowing—even wanting—people to die unnecessarily. There’s also been an outcry raised against the notion that anyone should be given a placebo in a prospective, double-blind, placebo-controlled randomized trial. Isn’t it morally and ethically wrong to deny someone a chance for a better outcome by giving them a sugar pill?
The answer is no. It’s our optimism bias that makes us think otherwise. When testing an intervention, everyone hopes it’s going to work. We often expect that it’s going to work. Further, we have better knowledge of what will happen if we don’t get the intervention—of the risk we have for experiencing a bad outcome. So it seems as if receiving a placebo is putting us at a disadvantage, dooming us to remain at the highest level of risk.
But this is an illusion. Because of our optimism bias, we don’t properly consider the real possibility that the intervention being tested may lead to worse outcomes. We’re further biased into ignoring this possibility by anecdotal observations that the intervention has helped others. (Usually, it’s these anecdotal observations that lead us to test the intervention in a properly designed trial in the first place.) Finally, we’re biased to ignore the possibility that the intervention may lead to worse outcomes by our knowledge of the mechanism by which the intervention might work. For example, we might know that a drug kills a virus in a petri dish in a lab.
But time and time again, when we test interventions that should work for all the reasons mentioned above and more, and we measure the outcomes that really matter—not, for example, how much a drug reduces viral load in a patient’s mouth but rather how much it reduces mortality—we discover that many interventions don’t work and some actually makes things worse. In fact, one study suggested that as many as 40 percent of commonly accepted medical practices, when finally subjected to properly designed studies, are found to not work.
That’s why we need to do properly designed trials, trials that are double-blinded so the biases of neither the subjects nor experimenters can influence the results, that are randomized so that all variables except the treatments given are roughly the same, and that include a placebo arm so that the subjects’ expectations don’t confound the outcomes. Without all of these design features, interventions can easily look like they work when they don’t. And though trial subjects are usually biased in favor of wanting to receive the intervention and not the placebo, if they really understood the likelihood of being helped compared to the likelihood of being harmed by the intervention, they might be wiser to want the placebo.
SHOULD WE WEAR MASKS?
A new study sheds more definitive light on this important question. The study looked at viral RNA shed in respiratory droplets (particles > 5 micrometers) and aerosols (particles < 5 micrometers) in infected subjects wearing surgical masks and not wearing surgical masks. Three different viruses were studied: seasonal coronavirus (not SARS-CoV-2 but perhaps similar), influenza, and rhinovirus (one cause of the common cold).
In the air around infected patients not wearing surgical masks, the investigators detected seasonal coronavirus RNA in respiratory droplets and aerosols in 30 percent and 40 percent of samples, respectively. In the air around infected patients wearing surgical masks, they detected no coronavirus RNA at all. The difference wasn’t quite statistically significant for respiratory droplets, but it was for aerosols. The participants were all coughing during the 30 minutes that the air around them was being sampled—except for 4 patients infected with seasonal coronavirus, who didn’t cough at all and around whom also no viral RNA was detected in either respiratory droplets or aerosols even in patients not wearing masks. Though this was a very small number, it suggests that patients infected with seasonal coronavirus aren’t likely to spread virus into the air by merely breathing.
One important caveat here is that the results were different for different viruses. For example, masks didn’t prevent the spread of rhinovirus into the air via respiratory droplets or aerosols at all. And masks only prevented the spread of influenza virus through respiratory droplets but not through aerosols. Thus, though seasonal coronavirus is likely similar in structure to SARS-CoV-2, we don’t know for certain that the two viruses spread into the air in the same way.
BOTTOM LINE: This was a well-designed study that strongly suggests if an infected patient wears a mask, he or she is much less likely to spread infectious virus into the air even when coughing. Seasonal coronavirus might not spread into the air at all through mere breathing, suggesting that asymptomatic spread of SARS-CoV-2, if structurally similar enough to seasonal coronavirus, may be occurring mostly via hand-to-face transmission. Wearing a mask to prevent asymptomatic spread of SARS-CoV-2 may not, therefore, be helpful. Then again, people may cough or sneeze for other reasons while asymptomatically infected with SARS-CoV-2 and inadvertently send infectious virus into the air. For that reason, wearing masks while out in public may still be prudent. Though currently the prevalence of asymptomatic SARS-CoV-2 infection is likely quite low (see below), as this prevalence increases, it may eventually become important for asymptomatic people to wear masks when going out in public.
WHO SHOULD GET ANTIBODY TESTED?
An antibody test isn’t typically used to determine if someone has an infection at the time they get the test. It’s used to determine if they had an infection sometime in the past. In general, a positive antibody test signifies immunity (though importantly, not always).
To decide if someone should get an antibody test to see if they’ve had COVID-19 or not, we first need to know the degree to which the antibody test is accurate. That is, we need to know the sensitivity and specificity of the test. These are statistical terms that have meanings apart from the ones found in the dictionary. Sensitivity is defined as the percentage of patients a test correctly identifies as having a disease, or the true positive rate. Specificity is defined as the percentage of patients a test correctly identifies as not having a disease, or the true negative rate.
We don’t yet know the sensitivity and specificity of any antibody test for SARS-CoV-2. (We’re not even sure we have a gold-standard with which to determine them, but the best we’re probably going to get is a positive RT-PCR test used in combination with COVID-19 symptoms in patients with typical chest CT findings.) But let’s assume we get a test with characteristics that are unusually good, say, 96 percent for sensitivity and 97 percent for specificity. That would mean that 96 percent of the time when a person has the disease, the test will correctly label that person as positive, and 97 percent of the time when a person doesn’t have the disease, the test will correctly label that person as negative. This also means that 4 percent of the time the test will tell us a person didn’t have the disease when he or she did (the false negative rate) and that 3 percent of the time the test will tell us a person did have the disease when he or she didn’t (the false positive rate).
These percentages sound pretty good, right? Well, they are. But there are two further issues we have to consider. The first is that sensitivity and specificity only tell you how likely it is that a test will correctly identify a disease as being present or absent in a population. It doesn’t tell an individual person how to interpret their own particular result. That is, if you test antibody positive for SARS-CoV-2, how likely is it that you had COVID-19? And if you test antibody negative, how likely is that you didn’t have COVID-19? People assume that if a test tells you that you’re positive, you can say with 100 percent certainty that you had the disease, and if a test tells you that you’re negative, you can say with 100 percent certainty you didn’t have the disease. But this isn’t how it works. How confident you can be about what a positive or negative test means for you varies. The confidence with which you can believe a positive test result is called the positive predictive value (PPV). The confidence with which you can believe a negative test result is called the negative predictive value (NPV). Those are the variables we really want to know when we get tested. For example, if an antibody test says you’re positive for SARS-CoV-2, but the likelihood of that positive being real—that is, the PPV—is only 50 percent, that test result is worthless. Same for a negative result.
The second problem is that the PPV and NPV aren’t determined only by the sensitivity and specificity of the test. They’re also determined the prevalence of the disease in the population you’re testing. In general, the more prevalent a disease is in a population, the better the PPV will be and therefore the more confident we can be that a positive test result is correct. So, in addition to knowing the sensitivity and specificity of a test, we also need to know the prevalence of the disease for which we’re testing.
HOW CAN WE ESTIMATE THE PREVALENCE OF COVID-19?
The COVID-19 outbreak onboard the Diamond Princess represents a closed population with which to understand how the SARS-CoV-2 virus might behave in the population at large. We can use the data gathered from the ship to estimate the prevalence of the disease on the ship and therefore potentially in society at large (the testing on board the ship was done in circumstances arguably more conducive to spread of the disease than currently exist in the world now, suggesting the prevalences onboard the ship may represent the worst we can expect from the disease). The total number of SARS-CoV-2 positives on board the Diamond Princess divided by the total population aboard the Diamond Princess—that is, the prevalence of the disease on the Diamond Princess—was 20.6 percent. A full 51 percent of these positives were asymptomatic (they didn’t just test people with symptoms; they tested everyone on board). Thus, it’s reasonable to assume that to estimate the prevalence of SARS-CoV-2 in the general population, we only need to double the number of SARS-CoV-2 positives in the population (because the number of symptomatic patients on board the Diamond Princess was half the total number of infections).
As of this writing (and it’s changing literally every day), the total number of symptomatic SARS-CoV-2 positives in the U.S. is 648K (out of 3.3M tested). Given the likelihood that the false negative rate of the RT-PCR test may be 26 percent among patients with infectious symptoms, we also need to take the total number of negative tests, 2.6M (= 3.3M – 648K), and multiply by 26 percent to identify the hidden number of symptomatic SARS-CoV-2 infections, which would be another 676K (= 2.6M x 0.26). Add that number (676K) to the number of symptomatic SARS-CoV-2 positives from above (648K) and we get a total of 1.3M total symptomatic COVID-19 patients in the U.S. as of this writing.
But we’re not done. This calculation leaves out the total number of people with infectious symptoms who have COVID-19 who haven’t been tested. Given how badly we’ve been under testing, this number is likely quite large. So we should say that the minimum prevalence of COVID-19 in the U.S. as of this writing is twice the number of known symptomatic SARS-CoV-2 positives, or 1.3M x 2 = 2.6M infected people in the U.S.
This suggests that the minimum prevalence of SARS-CoV-2 infection in the U.S. is 2.6M divided by the total U.S. population of 331M x 100, which equals 0.78 percent.
But what about that other group, those people with infectious symptoms who have COVID-19 who haven’t been tested? We don’t know how big that group is, but let’s assume it’s ten times the number of people with symptoms who’ve tested positive (and with symptoms who’ve falsely tested negative). That would be another 13M symptomatic, infected, untested people (= 1.3M x 10) who we’d need to add to 1.3M symptomatic, infected, tested people to make a total of 14.3M symptomatic, infected people in the U.S. as of this writing. Then double that number to include the number of asymptomatic infected people in the U.S. as of this writing to yield a total number of infections in the U.S. as of this writing of 28.6M people. That would yield a prevalence of 8.6 percent in the U.S.
BOTTOM LINE: The true prevalence of COVID-19 in the U.S. as of this writing—meaning your current likelihood of getting it—is probably somewhere between 0.78 percent and 8.6 percent.
Of course, that prevalence will also vary by region. If we do the calculation above for the Chicagoland area (Cook County), for example, the prevalence ends up being 7.3 percent (17.3K confirmed symptomatic cases + 173K unconfirmed symptomatic cases (17.3K x 10) = 190.3K total symptomatic cases + 190.3K asymptomatic cases = 380.6K total cases divided by the total cook county population of 5.15M x 100).
(By the way, it only took three days—the amount of time it took to move from the first draft of this post to its published version—for the maximum prevalence in the U.S. to move from 4.3 percent to 8.6 percent and for the prevalence in the Chicagoland area to move from 6.6 percent to 7.3 percent.)
Most importantly, that prevalence will also vary by symptom group. That is, if you’ve had infectious symptoms consistent with COVID-19, your likelihood of actually having had COVID-19 will be higher than if you haven’t had infectious symptoms consistent with COVID-19. We can calculate these two prevalences as follows: In the symptomatic group, the prevalence is the total number of people with infectious symptoms who have COVID-19 divided by the total number of people with infectious symptoms x 100. As of this writing, in the U.S., that would be 1.3M divided by 3.3M x 100, which yields a prevalence of 39 percent. (This prevalence will vary depending on exactly when you had symptoms. The more recent your symptoms, the higher the likelihood that they represented a COVID-19 infection.) In the asymptomatic group, the prevalence is the total number of people without infectious symptoms who have COVID-19 divided by the total number of people without infectious symptoms (that latter number is 331M – 14.3M = 317M). As of this writing, in the U.S., that would be 1.3M divided by 317M x 100, which yields a prevalence of 0.41 percent.
BOTTOM LINE: As of this writing, if you have had recent infectious symptoms consistent with COVID-19, the likelihood that you have (or had) COVID-19 is 39 percent. If you haven’t had infectious symptoms consistent with COVID-19, the likelihood that you have (or had) COVID-19 is 0.39 percent. If you’ve had infectious symptoms consistent with COVID-19 since January 2020, the likelihood that you had COVID-19 rests somewhere between 0.39 percent and 39 percent depending on when you were sick (the more distant the symptoms, the less likely they were from COVID-19).
With these guesses about the current prevalences of COVID-19 in these different groups, we can now calculate the PPV and NPV of an antibody test that has the outstanding sensitivity and specificity we assumed above—that is, a sensitivity 96 percent and a specificity of 97 percent. If we assume a population sample of 1000 people, we can work backward from the sensitivity and specificity to fill in the following cells with the data from above for each prevalence:
COVID-19 infection present | COVID-19 infection absent | Predictive Values | |
---|---|---|---|
(+) antibody test | True positive rate | False positive rate | PPV |
(-) antibody test | False negative rate | True negative rate | NPV |
Test characteristics | Sensitivity=96% | Specificity=97% |
For people who have had infectious symptoms since January 2020 (prevalence of at most 39 percent), the antibody test we’re considering would be expected to yield the following results:
COVID-19 infection present (390 people) | COVID-19 infection absent (610 people) | Predictive Values | |
---|---|---|---|
(+) antibody test | True positive=374.4 people | False positive=18.3 people | PPV=95.3% |
(-) antibody test | False negative=15.6 people | True negative=591.7 people | NPV=97.4% |
Test characteristics | Sensitivity=96% | Specificity=97% |
For people who haven’t had infectious symptoms since January 2020 (prevalence of at most 0.41 percent), the antibody test we’re considering would be expected to yield the following results:
COVID-19 infection present (4.1 people) | COVID-19 infection absent (995.9 people) | Predictive Values | |
---|---|---|---|
(+) antibody test | True positive=3.9 people | False positive=29.9 people | PPV=11.5% |
(-) antibody test | False negative=0.2 people | True negative=966 people | NPV=99.9% |
Test characteristics | Sensitivity=96% | Specificity=97% |
The thing to notice here is that, even when the sensitivity and specificity of a test don’t change, the positive predictive value (PPV) and negative predictive value (NPV) change with the prevalence of the disease (PPV of 95.3 percent vs. 11.5 percent and an NPV of 97.4 percent vs. 99.9 percent). When the disease is even moderately prevalent in the population you’re testing, if the test’s sensitivity and specificity are high enough, the PPV and NPV are good enough that you can rely on the test. But even with a high sensitivity and specificity, if the disease has a low prevalence in the population you’re testing, you can see how the PPV is worthless—meaning that a positive test tells you nothing.
CONCLUSION: From these calculations, we finally have our answer: If you’ve had infectious symptoms since January 2020 and you get an antibody test with a high enough sensitivity and specificity, you can believe the results of the test, whether positive or negative. Once we have an antibody test available with good sensitivity and specificity here at ImagineMD, we’ll begin testing patients who give a history of having had symptoms consistent with COVID-19. If you haven’t had infectious symptoms since January 2020, getting a positive antibody test will tell you nothing. Getting a negative antibody test means you almost certainly haven’t had COVID-19—but given that you never had symptoms, you could already assume that. Until the prevalence of COVID-19 dramatically escalates, we’re recommending against testing patients who’ve never been symptomatic.
The one good piece of news is that, as the prevalence of COVID-19 infection, both symptomatic and asymptomatic, inevitably rises in the population, the PPV for asymptomatic infections will improve. When enough people in the population have been infected, testing asymptomatic people might begin to make sense—if the sensitivity and specificity of the available test are high enough.
But none of this answers the question we really need to answer: what degree of antibody positivity confers immunity and for how long? Right now, a positive antibody test that you believe is accurate would only confirm that you’ve had the disease. It won’t tell you if you’re immune to getting it again and can therefore go back to work safely.
To answer this question with any kind of scientific rigor will take time—time for people proven to have antibodies to be observed in comparison to a group proven not to have antibodies to see if the infectivity rates differ over time. Because it’s likely we can’t—or won’t—wait to reopen the economy before we can prove that antibodies to SARS-CoV-2 provide immunity to COVID-19, we’re almost certainly going to have to make the call with imperfect information. And unfortunately there’s a chance that antibodies to SARS-CoV-2 don’t confer immunity—or don’t confer it for long—because in children, reinfection has been shown to occur with other types of coronaviruses, in some cases within months. Animal studies may help us answer this question, but the BOTTOM LINE is that when people who are proven to have antibodies to SARS-CoV-2 initially go back out into the workforce and the world, they’ll be making a bet. Possibly a good bet, but a bet nonetheless.
- For previous posts related to COVID-19, see:
- Coronavirus February 2020—Part 1 What We Know So Far
- Coronavirus March 2020—Part 2 Measures to Protect Yourself
- Supporting Employee Health During the Coronavirus Pandemic
- Coronavirus March 2020—Part 3 Symptoms and Risks
- Coronavirus March 2020—Part 4 The Truth about Hydroxychloroquine
- Coronavirus April 2020—Part 5 The Real Risk of Death
[jetpack_subscription_form title=” subscribe_text=’Sign up to get notified when a new blog post has been published.’ subscribe_button=’Sign Me Up’ show_subscribers_total=’0′]
As per usual, great writing/research. Something that has concerned me from the beginning is the tremendous push back from public health officers on mitigation, e.g. masks, when by their own admission so little was understood about this novel virus. Instead of, as you would expect, erring on the side of caution, public health erred on the side of risk, shaming and shunning the public prophylactic use of masks; citing unsupported anecdotal claims, i.e., touching face, etc. While this may have been due to a profound lack of government preparedness and supply, to continue for weeks and months in this regard was reckless. Public health has even gone so far as making trade in these materials illegal, instead suggesting to the public to reuse or even in CDC official policy to produce DIY protective/mitigation equipment. Science fiction writers wouldn’t dare write something so absurd, fearing this actual policy would be so unbelievable…
Troy, agreed.
Mentioning that we have several N95 masks in a partially used box in our basement (leftover from home repairs) has become akin to admitting illegal drug use or gun ownership.
I and my loved ones wear a N95 when we must enter the grocery store or pharmacy.
I believe that if one mask stops me from landing in the hospital, it has prevented 200+ masks from being consumed there, nursing me back to health.
“But we’re not done. This calculation leaves out the total number of people with infectious symptoms who have COVID-19 who haven’t been tested. Given how badly we’ve been under testing, this number is likely quite large. So we should say that the minimum prevalence of COVID-19 in the U.S. as of this writing is twice the number of known symptomatic SARS-CoV-2 positives, or 1.3M x 2 = 2.6M infected people in the U.S.
This suggests that the minimum prevalence of SARS-CoV-2 infection in the U.S. is 2.6M divided by the total U.S. population of 331M x 100, which equals 0.78 percent.
But what about those symptomatic people who haven’t been tested yet who have COVID-19 nonetheless? We don’t know that number, but let’s assume it’s ten times the number of people with symptoms who’ve tested positive (and with symptoms who’ve falsely tested negative). That would be another 13M symptomatic, infected, untested people (= 1.3M x 10) who we’d need to add to 1.3M symptomatic, infected, tested people to make a total of 14.3M symptomatic, infected people in the U.S. as of this writing. Then double that number to include the number of asymptomatic infected people in the U.S. as of this writing to yield a total number of infections in the U.S. as of this writing of 28.6M people. That would yield a prevalence of 8.6 percent in the U.S.”
I’ve read this several times and somehow the distinction between these 2 groups (first paragraph “This calculation leaves out the total number of people with infectious symptoms who have COVID-19 who haven’t been tested”) and (second paragraph “But what about those symptomatic people who haven’t been tested yet who have COVID-19 nonetheless?”) escapes me.
Thanks
What a fantastic post! I’m guessing from your post that hoping for antibody tests with sensitivity and specificity of 96% and 97%, respectively, is probably not realistic at this point, but what then is realistic? And what minimum sensitivity and specificity levels are you looking for in a test that you would consider worthwhile for use in your practice (or are those levels moving targets that depend upon prevalence)?